
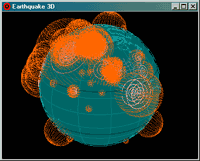
Mitigating the damaging level of earthquake hazards has been a long endeavor in seismology 1, 2, 3. After receiving data, the network takes less than two hundred milliseconds for predicting the source focal mechanism reliably on a single CPU. The network learns the global waveform characteristics from theoretical data, thereby allowing the extensive applications of the proposed method to regions of potential seismic hazards with or without historical earthquake data. The FMNet trained with 787,320 synthetic samples successfully estimates the focal mechanisms of four 2019 Ridgecrest earthquakes with magnitude larger than Mw 5.4. Here we propose a novel deep learning method namely Focal Mechanism Network (FMNet) to address this problem. Advanced technologies such as Artificial Intelligence (AI) has been introduced to solve various problems in real-time seismology, but the real-time source focal mechanism is still a challenge.
#Real 3d earthquake information full
An immediate report of the source focal mechanism with full automation after a destructive earthquake is crucial for timely characterizing the faulting geometry, evaluating the stress perturbation, and assessing the aftershock patterns.
